Introduction
Breast cancer is the leading cause of cancer incidence globally, affecting approximately 2.3 million people (11.7% of all cancers) and resulting in 670,000 deaths (6.9% of cancer-related fatalities) in 2022. It ranks as the most diagnosed cancer in many countries and is the leading cause of cancer-related death in 110 nations. Notably, it remains the most frequently diagnosed cancer among women. Curious about the numbers? In the USA alone, an estimated 310,720 new cases of invasive breast cancer are expected to be diagnosed in 2024, with around 42,250 cases leading to death—accounting for 15% of total cancer-related deaths. This highlights the ongoing significance of breast cancer as both a prevalent diagnosis and a major health concern for women. Now, let’s talk about progress! Over the past 30 years, population-based breast cancer screening has been vital in early detection, contributing to a remarkable 40% drop-in breast cancer mortality rates from 1989 to 2017. This reduction translates to approximately 375,900 lives saved! However, while the mortality rate is still decreasing, the pace has slowed—falling from 1.9% per year (1998-2011) to 1.3% per year (2011-2017) and risen to 3.4% in 2019. So, what has fuelled this progress? Advances in medical imaging technology and a deeper understanding of the complex biological and chemical characteristics of breast cancer have played a significant role. Yet, the journey of managing breast cancer remains intricate and challenging.
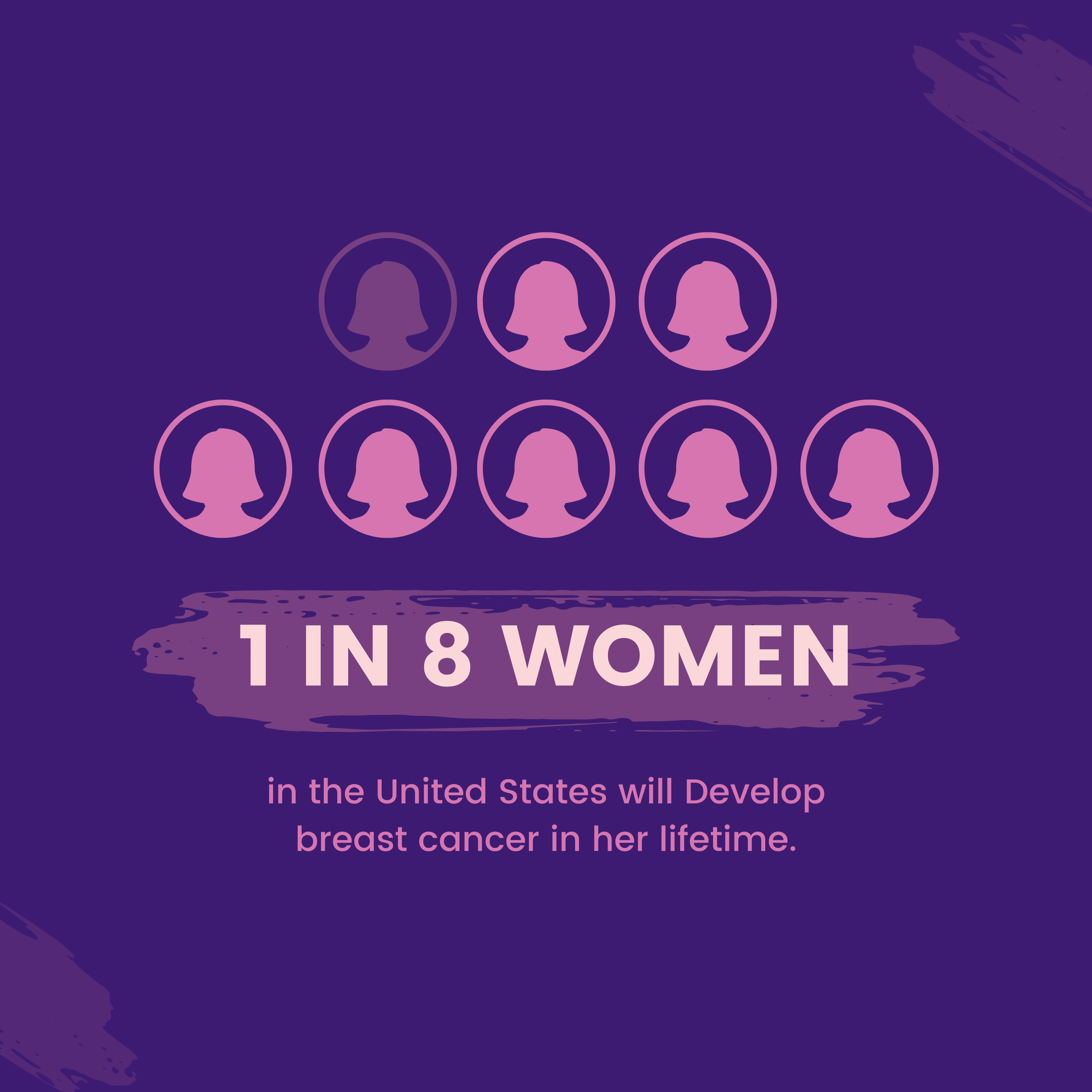
Artificial Intelligence (AI) is rapidly transforming various industries, enhancing productivity, efficiency, and accuracy in ways we never thought possible. But what does this mean for healthcare, specifically in breast cancer diagnosis? According to the National Breast Cancer Foundation's 2020 report, AI has played a pivotal role in diagnosing over 276,000 cases of breast cancer. Additionally, 48,000 cases—especially those involving aggressive cancer types—were identified using non-deep learning methods. This is more than just impressive numbers; it reflects a groundbreaking shift in how we approach cancer detection.
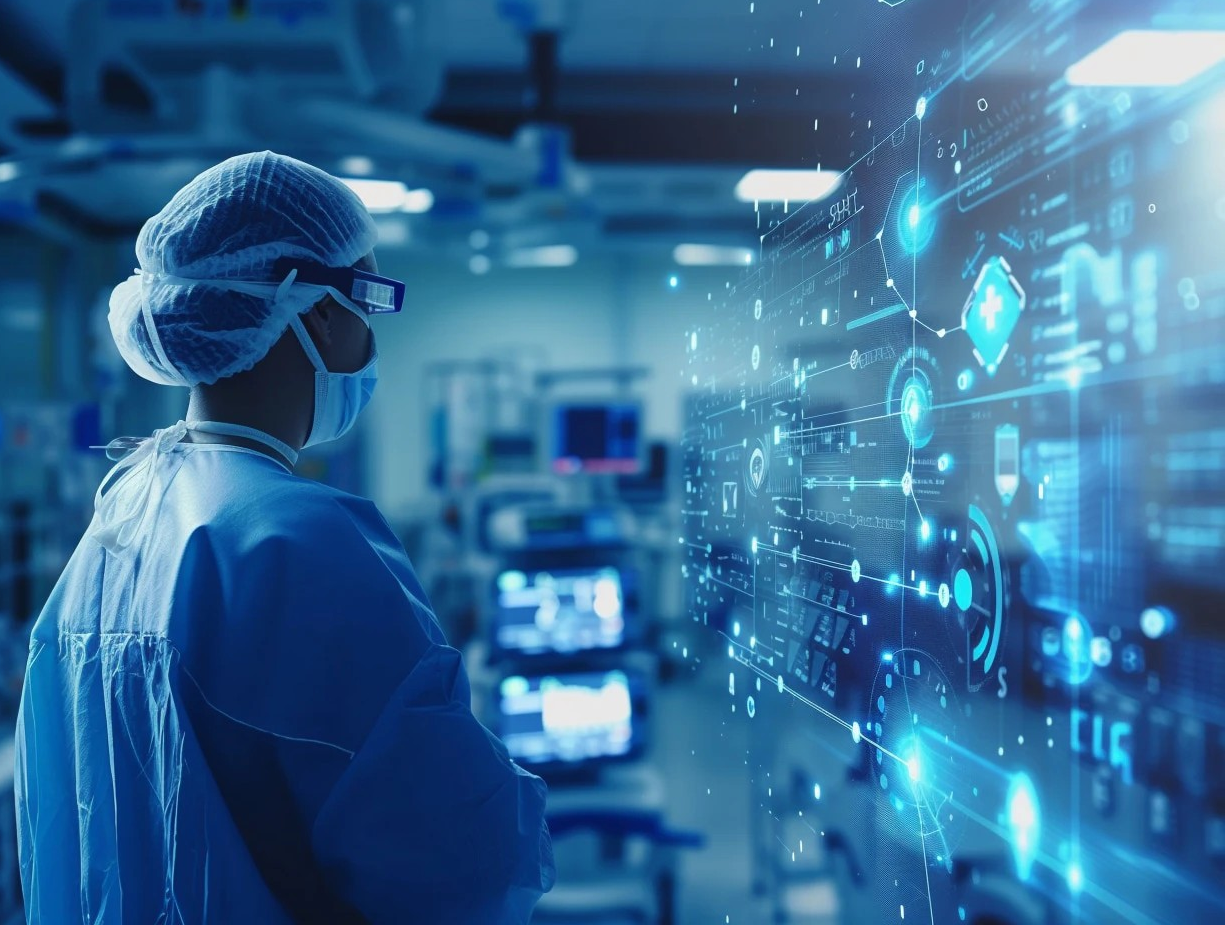
AI is one of the most exciting developments in science and medicine today. Its capacity to process vast datasets, identify patterns, and make precise predictions holds tremendous promise for earlier cancer detection, tailored treatment plans, accelerated drug discovery, and ultimately, improved patient outcomes. Among these applications, AI’s most profound impact is seen in the analysis of complex medical images. It provides valuable data for quantitative assessments while significantly reducing the radiation risk typically associated with breast imaging. By mimicking human decision-making with operational precision, AI demonstrates a level of "superintelligence," allowing it to rapidly incorporate feature learning, process complex multidimensional data, and utilise diagnostic data from various clinical trials. Recognising these advancements, medical professionals, researchers, and oncologists are increasingly aware of AI's significant potential in breast cancer diagnosis.
Integration of AI in Breast Cancer Screening and Diagnostics
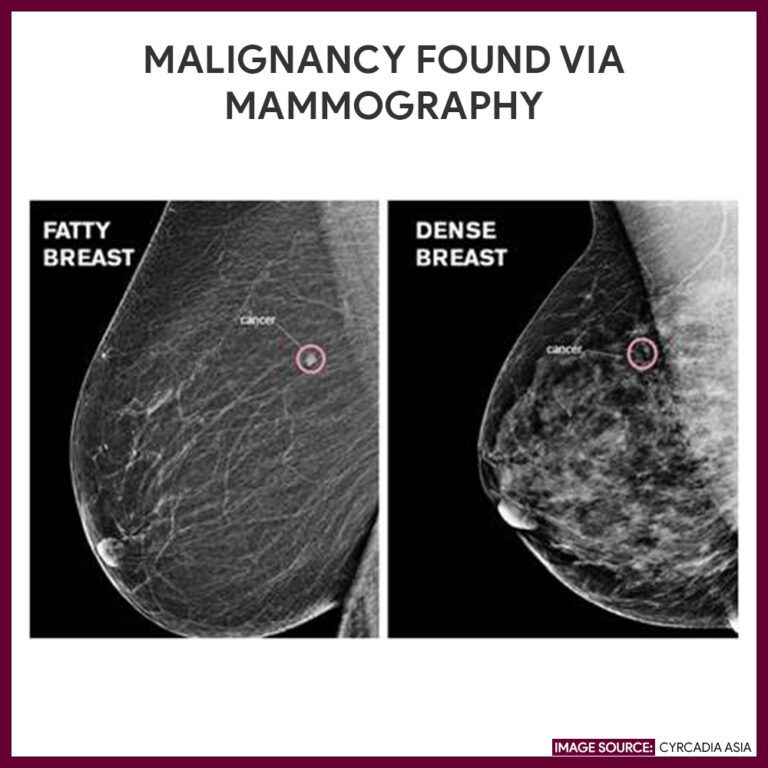
AI is emerging as a powerful tool in the early and precise detection of breast cancer, which is crucial for improving patient outcomes and saving lives. Over the last three decades, breast cancer deaths have decreased by 43%, largely due to advancements in screening and treatment methods. Regular screenings, particularly mammography, are the most effective means of early detection, enabling healthcare professionals to identify cancer when it is still small and localised. When breast cancer is detected early, patients have a significantly higher chance of successful treatment and may be able to avoid aggressive therapies. Mammography has long been regarded as the gold standard in breast cancer screening, effectively spotting abnormalities in breast tissue before any physical symptoms, such as lumps, manifest.
However, mammograms have their limitations. Some women—especially those with dense breast tissue or specific risk factors like gene mutations or family history—may require additional imaging techniques, such as ultrasound or MRI. Additionally, false positives can lead to unnecessary callbacks and biopsies, causing undue stress for patients. One noteworthy AI tool making strides in this area is GALEN Breast. This innovative tool analyses breast biopsy samples to detect cancer cells and classify them into different types of breast cancer. In tests using extensive datasets from multiple institutions, GALEN Breast achieved impressive accuracy rates: 0.99 (99%) for detecting invasive carcinoma and 0.98 (98%) for ductal carcinoma in situ (DCIS).
Despite the challenges that exist, mammography and other screening technologies continue to save lives. Ongoing research focused on improving the accuracy of these screening methods and diagnostic pathology is essential, and AI is poised to play a significant role in advancing this field.
Improving breast imaging with AI
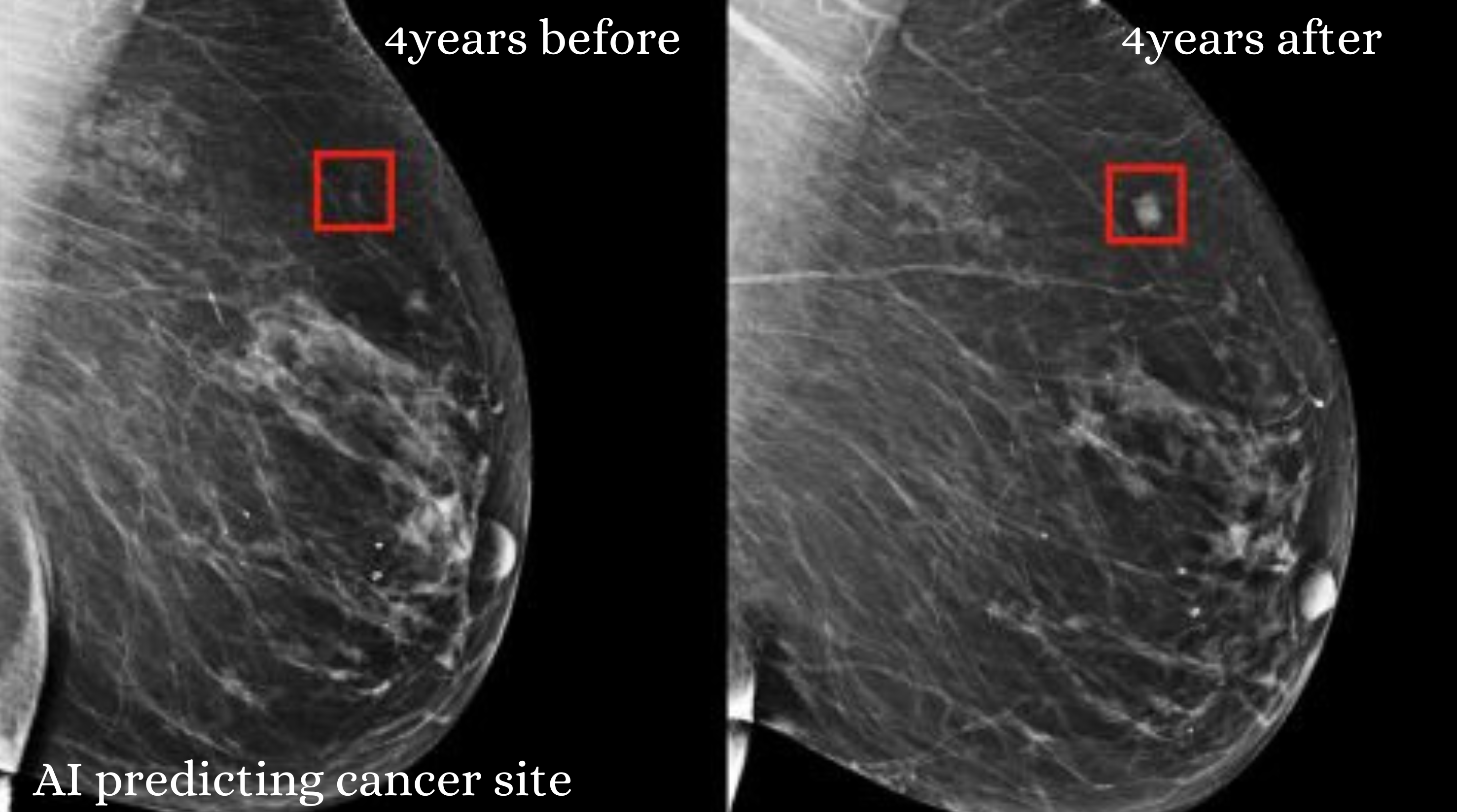
Highly skilled radiologists play a crucial role in interpreting breast images, combining their expertise in selecting appropriate imaging techniques with a thorough assessment of each patient's anatomy. When a woman undergoes a mammogram or other screening, her radiologist meticulously describes findings, detailing aspects such as location, size, shape, density, and other relevant features of any abnormalities or pathologies.
AI is transforming this workflow, making it more efficient and providing quantitative analyses that minimise human bias. AI algorithms can automate the interpretation of mammograms, ultrasounds, and MRI scans, allowing patients to receive their results more quickly. AI techniques are particularly beneficial in detecting breast cancer at early stages, often identifying cases that may have gone unnoticed. Methods like image enhancement and de-noising help improve the clarity of breast images, allowing radiologists to visualise anatomical structures more clearly. This capability enables AI-powered tools to detect subtle abnormalities, clarify ambiguous features, and recognise patterns that may not be readily apparent to the human eye. Additionally, AI can estimate tumour size and shape, enhancing the accuracy of screenings and reducing both false positives and negatives.
Moreover, AI-powered breast imaging has the potential to improve breast cancer care in low-resource or rural areas, where access to specialists may be limited. These AI systems allow for remote interpretation of imaging, ensuring timely diagnosis and treatment for patients, regardless of their location. Given that mammography is currently the most time- and cost-effective screening method, enhancing its accuracy can lead to fewer follow-up visits, unnecessary biopsies, and more expensive tests like MRIs.
The collaboration between AI and radiomics presents immense possibilities for advancing breast cancer care, ultimately leading to improved outcomes for patients. By leveraging AI's capabilities alongside the expertise of radiologists, the future of breast cancer detection looks promising.
AI in early detection
AI research is revolutionising breast cancer imaging and diagnosis, often utilising advanced models that surpass traditional screening methods in detecting cancer. Deep learning (DL) algorithms, which simulate brain functions, have proven to be more effective than traditional machine learning (ML) methods in analysing breast cancer images. These improvements significantly reduce false positives (incorrect cancer detections) by employing Support Vector Machine (SVM) classifiers, which sort data to enhance accuracy.
Traditional mammography can sometimes overlook cancers due to challenges such as breast density, where denser tissue complicates detection, and the small size of tumours. However, when paired with digital mammography, AI excels in identifying asymmetry and distortion (irregularities in breast shape). Despite the absence of extensive public databases for AI training, breast lesion detection software has made substantial progress. AI can now automatically locate lesions in images, achieving accuracy comparable to that of experienced radiologists. Convolutional Neural Networks (CNNs), specifically designed for image analysis, efficiently identify lesion boundaries and perform these tasks faster than human counterparts. U-nets, a particular type of CNN, are frequently used to differentiate areas in mammography images and can distinguish between various tissue types, such as glandular (related to glands) and adipose (fat) tissue.
Manual classification by radiologists can be time-consuming and inconsistent, which highlights the importance of early detection in breast cancer treatment. The National Breast Cancer Foundation emphasises that identifying breast changes early, while the cancer is still localised, leads to a remarkable 99% five-year survival rate. Regular self-examinations and mammograms are vital in improving these survival rates, reinforcing the critical role of both AI advancements and routine screening in breast cancer care.
AI for breast cancer risk assessment and genetic alteration prediction
Mammographic density, as assessed by the Breast Imaging Reporting and Data System (BI-RADS), is a significant risk factor for breast cancer. Recent screening methods, such as those tested in the Dense Tissue and Early Breast Neoplasm Screening trials, have begun to incorporate breast density into evaluations of cancer risk for women. However, the current measurement techniques primarily depend on the subjective judgments of radiologists, which can lead to considerable variability. This inconsistency stresses the need for more reliable and standardised methods to enhance screening accuracy.
Tools like Volpara have been developed to address these challenges. Volpara automates the calculation of breast density as a percentage, yielding more consistent results across patients. Additionally, AI models trained on data from radiologists can provide automated, standardised measurements of breast density. These AI tools not only assist in assessing cancer risk but also help predict how well high-risk patients may respond to treatments. More research is needed to identify the most effective tools and to establish how they can be integrated into routine clinical practice. Traditional risk models, such as the Tyrer-Cuzick model, already consider breast density as a risk factor. AI-enhanced models are now improving the predictive accuracy of these assessments. A study by Arasu et al. demonstrated that AI models outperformed the Breast Cancer Surveillance Consortium (BCSC) model in predicting the likelihood of developing breast cancer over five years.
Moreover, AI has the capability to identify patterns in breast tissue that radiologists might overlook. For instance, Kim et al. developed a model that detects specific tissue patterns in high-risk individuals, enabling it to predict cancer risk even when analysing images from healthy breasts. Other notable AI models, such as MIRAI, have improved performance in predicting five-year breast cancer risk. At the same time, ProFound AI assists radiologists in enhancing cancer detection in digital breast tomosynthesis (DBT) images. These advancements highlight how AI can help personalise screening methods and frequencies based on individual risk factors, ultimately improving breast cancer detection and outcomes.
Where are the gaps?
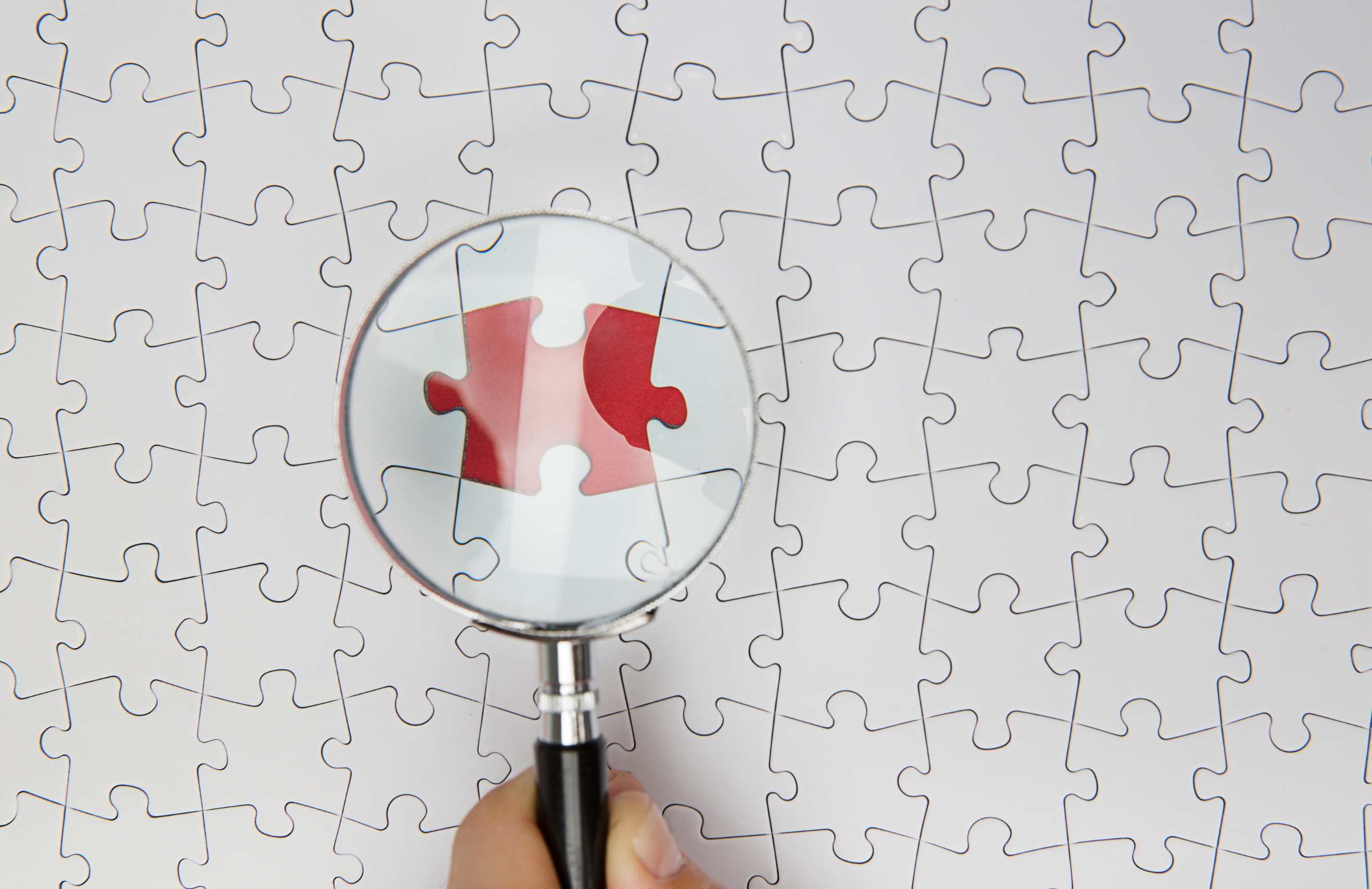
Age-Based Screening Limitations
Have you ever wondered why traditional mammography guidelines primarily recommend screening for women aged 40 and above? With an increasing number of younger women being diagnosed with breast cancer, this approach may not effectively catch early detections in this demographic. What if AI could help shift the focus from age-based to risk-based screening? By incorporating factors like genetics, lifestyle, and family history, personalised AI-driven screening schedules could significantly improve early detection for younger women. However, the reality is that such personalised approaches are not yet widely implemented. How can we advocate for these changes? Consider this: while AI has made mammograms more accurate, its potential to identify high-risk individuals for earlier or more frequent screenings remains largely untapped. Imagine a system where continuous risk assessments are possible, analysing factors such as genetics and personal history. This dynamic, personalised approach could revolutionise screening for those at higher risk. So, how do we bridge this gap in risk assessment?
Predicting Recurrence
AI has made significant strides in detecting primary breast cancers, but what about its ability to predict recurrence? Current models face challenges in quantifying recurrence risk based on previous cancer characteristics, such as tumour size and treatment response. What if we could enhance AI systems to incorporate more robust recurrence prediction algorithms using imaging and clinical data? This could dramatically improve long-term patient care. How can we drive research in this direction?
Actionability of Early Detection
One of AI’s impressive strengths is its ability to detect cancerous changes before they are visible on traditional mammograms. Yet, there is a critical gap: how do we translate this early detection into immediate clinical action? Without clear guidelines for addressing AI-detected anomalies, the potential for timely interventions may diminish. How can we establish protocols to ensure that early detections lead to effective action?
Data Diversity, Quality, and Long-Term Tracking
AI models thrive on large, diverse, and high-quality datasets. But what happens when there’s a lack of diversity in the data? This limitation can hinder AI’s ability to generalise across different populations. Moreover, many AI models lack access to longitudinal data, which is crucial for tracking patient outcomes over time. Addressing these gaps is vital for improving AI performance and ensuring accurate predictions regarding recurrence and long-term outcomes. What steps can we take to enhance data diversity and quality?
Integration into Clinical Workflows and Transparency
Integrating AI tools into clinical workflows is another significant challenge. How user-friendly are these AI predictions for healthcare providers? Collaboration between AI developers and healthcare professionals is essential to create tools that are not only easy to use but also provide actionable insights. Additionally, AI models often function as “black boxes,” making it challenging for clinicians to understand the basis of predictions. How can we improve transparency and ensure that clinicians can trust these tools?
Regulatory and Ethical Concerns
Lastly, the use of AI in healthcare raises essential regulatory and ethical issues. Questions about patient privacy, data security, and the potential for algorithmic bias are at the forefront of this discussion. How can we address these concerns to ensure the responsible use of AI in breast cancer screening and recurrence prediction? AI models must comply with ethical standards to protect patient rights and maintain public trust. What measures should we advocate for to ensure ethical AI use in healthcare?
Conclusion
As we stand at the crossroads of technology and medicine, the integration of AI into breast cancer detection and management offers unprecedented opportunities to transform patient care. By addressing the existing gaps in screening, risk assessment, and data quality, we can harness the power of AI to tailor personalised solutions that not only enhance early detection but also improve treatment outcomes.
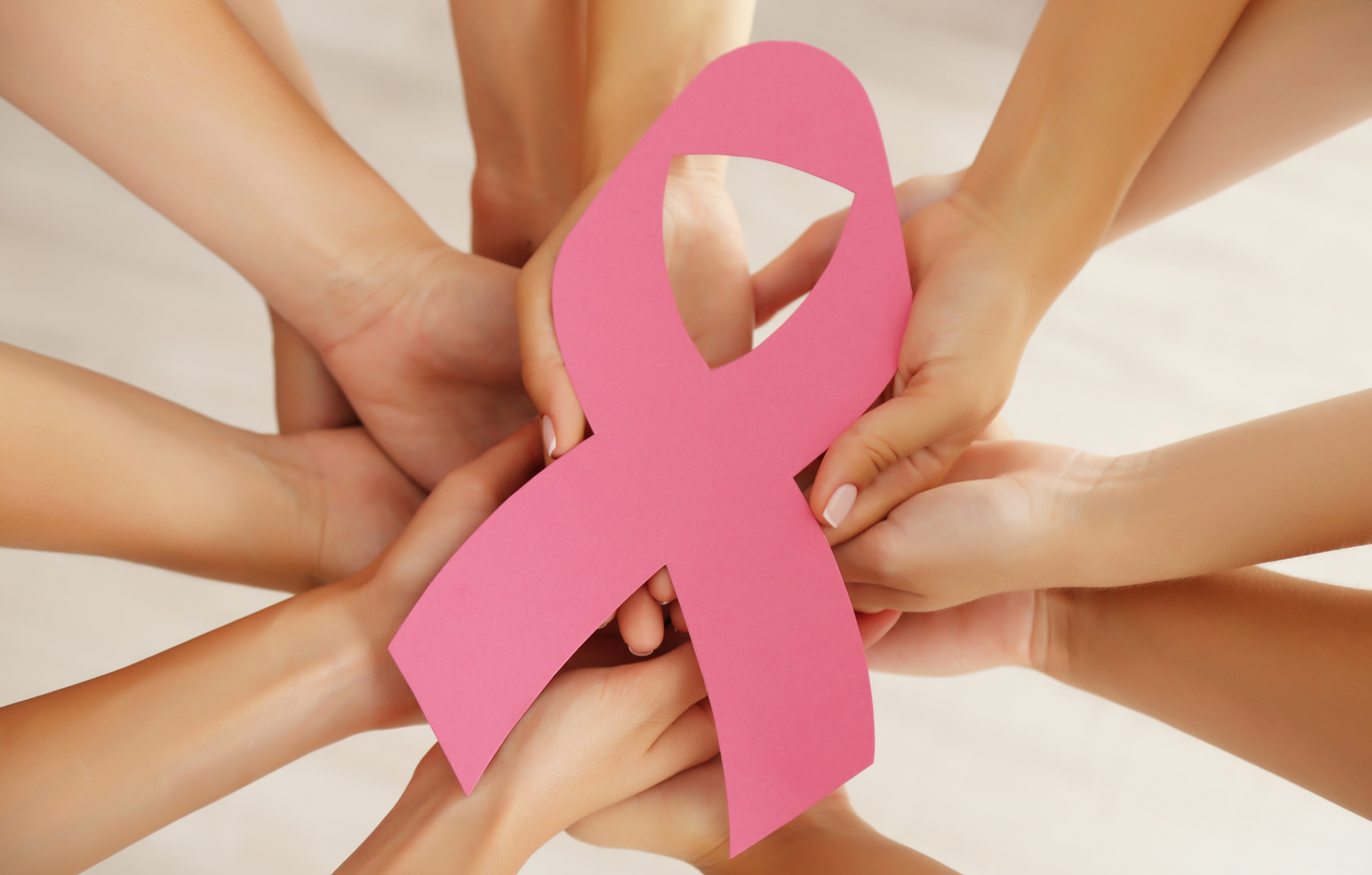
The journey ahead is filled with challenges, but together, we have the potential to redefine breast cancer care, ensuring that no woman’s health is overlooked. After all, in the fight against breast cancer, every advancement counts, and every voice matters. Together, we can inform the path toward a healthier future for all.